(B01-3)
Natural science to date has been developed by extracting laws from natural phenomena by means of experiments, and by deriving fundamental laws in theoretical ways. In addition, simulations using computers have enabled the analysis of complex theoretical models, and we have been using the combination of these three methods to understand natural phenomena. However, recent technological developments in experiments and measurements, which have made it possible to obtain high-dimensional data, mean it is no longer easy to organize natural phenomena, and it is now difficult to identify the point at which we combine these three methods.
In this , the goal is to establish a universal modeling principle that extracts laws of nature by applying sparse modeling to extract important factors from high-dimensional natural-scientific data, by clarifying the analysis target, and by searching for a contact point between experiments and theory/simulations with physical modeling.
While effective methods of analysis for high-dimensional experimental data are desired as explained above, simulations of theoretical models have increased their size, as represented by all-atom simulations, and it is now difficult to compare and examine obtained high-dimensional data through experiments (Figure 1). Multivariate analysis of experimental and simulation data has been attempted; however, the current state is that each analytical method is investigated individually. We have been using estimates based on Bayesian statistics to analyze data, and we have recognized that the needs in research methods for natural science are not only to extract important factors from data, but also to understand from theoretical models the meanings of the extracted important factors and the relationships among them. We feel that it is an essential research subject to establish a universal modeling principle for the method of data-driven science, based on sparse modeling. This research aims to establish a systematic scheme to extract important factors from high-dimensional data by means of sparse modeling. This scheme will eventually enable feedback from experiments to theoretical models.
The purpose of this , which is based on sparse modeling, is to establish a universal modeling principle driven by spatiotemporal data for the extraction of laws of nature. To do this, we feel that it is important to present concretely typical examples of research methods, and we will conduct research mainly focusing on the three research subjects outlined below, which correspond to the extraction of laws of spatial structure formation, the extraction of dynamics of time evolution, and the extraction of spatiotemporal dynamics that merges those, respectively.
- [Subject 1] Sparse modeling of scanning tunneling microscopy (STM)
STM is an experimental technique to observe directly the surface of a material at the atomic scale. We will develop a modeling method to extract laws of nature that govern the spatial structure of the surface material from the obtained image data. In this subject, by applying sparse modeling to both experimental and simulation results, we will compare the extracted important factors, and aim to reconstruct a theoretical model. By doing this, we will show a typical example that realizes the concept shown in Figure 1, and we will establish the basis of methods of image data analysis for natural science. - [Subject 2] Sparse modeling of calcium imaging
By using measurement techniques, such as calcium imaging, it is now possible to directly observe spatiotemporal responses of neural activity in multiple neurons within neuronal circuits. In this subject, applying sparse modeling to imaging data, we will extract the dynamics of neural elements and the interaction among the elements as important factors, and we will develop a method to estimate the spatiotemporal dynamics of the neural system. Furthermore, by formulating a theoretical model that includes physical processes and by applying sparse modeling to simulation data, we will develop a method to establish a theoretical model from comparisons of important factors extracted from imaging and simulation data. - [Subject 3] Sparse modeling of dynamics of petrogenesis
The methodologies established in Subject 1 and Subject 2 are expected to be applied to various problems in natural science. In particular, we will focus on a problem of petrogenesis, and we will apply those methodologies to the extraction of laws of nature that govern the dynamics of petrogenesis. We will extract chemical compositions that significantly reflect the process of rock formation as important factors by applying sparse modeling to experimental data. At the same time, applying sparse modeling to simulations of a theoretical model that contains reaction and diffusion processes, we will clarify the details of the physical processes.
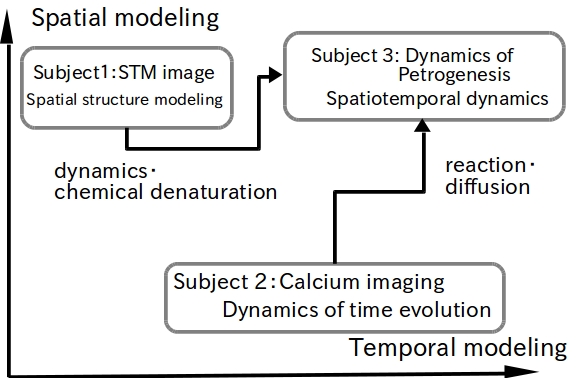