A never-ending series of improvements to measurement techniques has led to the ongoing creation of large quantities of high-dimensional observation data every day. While detailed data-centric science is promoted in fields such as bioinformatics, situations have been encountered where high-dimensional data analysis methods used in the field of astronomy also work effectively at completely different scales of magnitude in the life sciences (Science, Feb. 11, 2011). In this way, the introduction of diverse viewpoints becomes a driving force for the creation of innovative developments in various different situations.
In this , we are focusing on sparse modeling as a methodology for causing innovative developments through the incorporation of a wide variety of viewpoints. As shown in the lower part of the figure to the right, by collaborating organically with information science researchers and researchers performing experiments and measurements in a wide range of secondary science fields such as life science and geoscience, we will create a new academic field of high-dimensional data-drive science where latent regularities are unearthed from high-dimensional data.
As demonstrated by the launch of the Science Council of Japan's large-scale research project ("An innovative algorithmic foundation for the implementation of e-science"), the era of data-driven science is now truly upon us. Research into the foundations and applications of processing large amounts of data by advanced information science and technology are actively under way both in Japan and in other countries. Most of these studies are chiefly aimed at increasing the availability of information to users by building databases, developing software and so on. On the other hand, projects aimed at extracting scientific knowledge are still at the stage of searching for a specific approach to algorithms and research.
We are considering the use of sparse modeling as a general framework for extracting scientific knowledge from high-dimensional data. Since the mid 2000s, a lot of attention has been drawn towards an innovative technique for information extraction by sparse modeling called compressed sensing (CS), which can be used in a wide range of fields such as measurement engineering, communications engineering, medical engineering and biochemistry.
By conducting research together with experimental researchers in fields such as earth science and brain science, Masato Okada has developed a general sparse modeling method that extracts latent structures and laws from high-dimensional data of completely different types, such as tsunami deposits and nerve cells (see figure on right). From these successes, we have arrived at the concept of developing an innovative methodology of natural exploration by not only conducting joint studies with information science and individual fields of natural science, but also establishing close partnerships between information science and a wide range of different natural science fields.
The participants in this project include experimental and measurement researchers from a wide variety of fields in addition to information scientists. To exploit these benefits, a promotes the entire project to encourage close collaboration between project members and, individual achievements are promoted across the entire project to create high-dimensional data-driven science through the establishment of modeling principles. In addition to promoting the experimental and measurement fields by the introduction of data analysis techniques, this also leads to the formation of a mathematical foundation for high-dimensional data-driven science. With regard to these support initiatives and publicity initiatives, the following three subjects need to be considered.
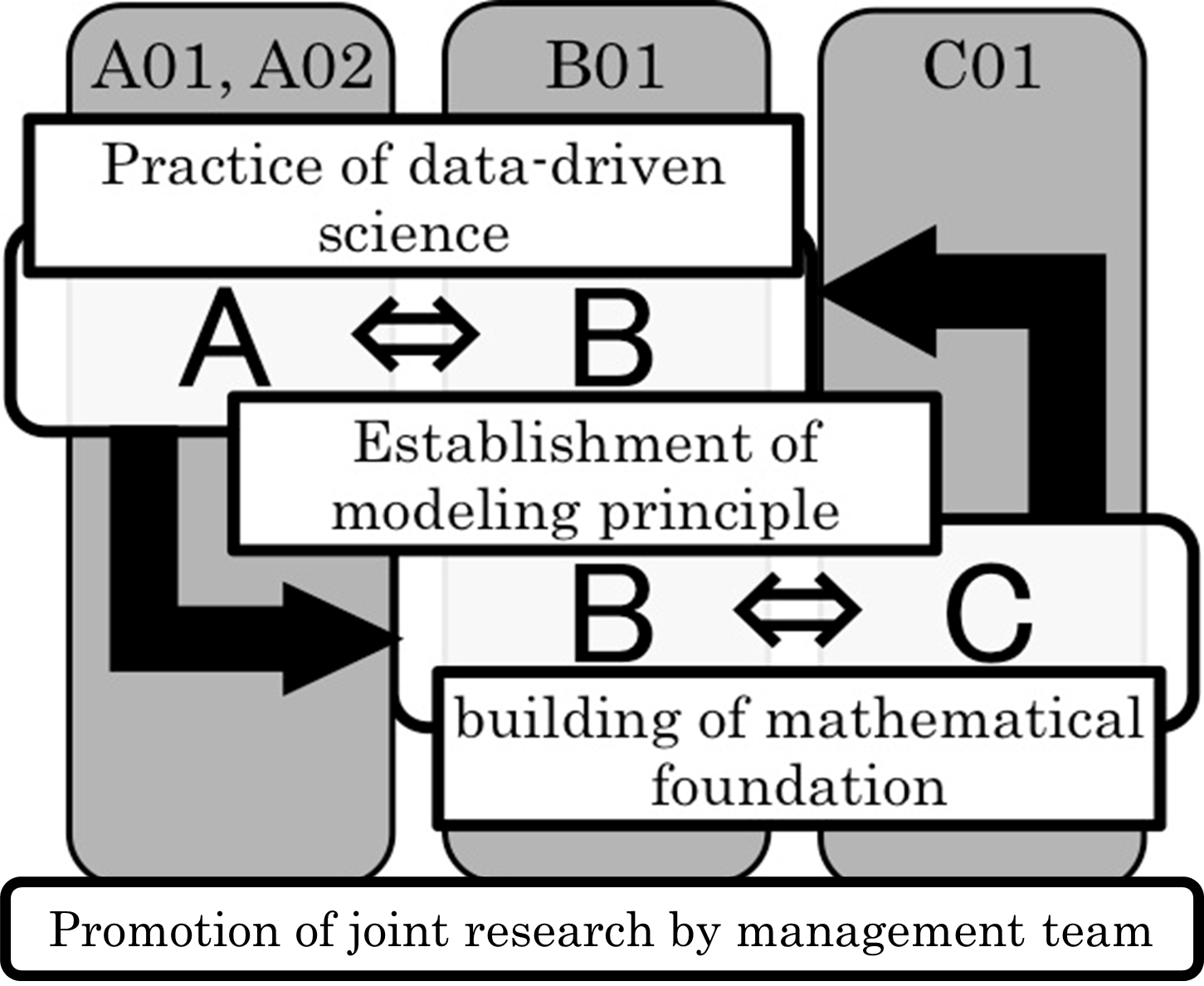
A number of people were appointed from the (A01, A02) and the (B01) to regulate the progress of research between each planning study, and to promote organic collaboration between information science and the natural sciences, which is the purpose of this applied research.
Sparse modeling is a type of data compression (source coding). The fusion of sparse modeling with the advanced mathematical information science of the (C01) leads to the construction of coding theory that can adapt flexibly to situations in diverse fields of natural science.
To ensure the training of the next generation of core researchers in high-dimensional data-driven science, we support seminars for young researchers that feature advocates of joint research, and we support joint studies and seminar presentations to young researchers at research institutes both in Japan and overseas.